Maximum entropy inference and facial attractiveness
Speaker: Miguel Ibàñez Berganza (Sapienza)
Title: Maximum entropy inference and facial attractiveness
Abstract - Facial attractiveness has recently been subject of an intense research in the machine learning community, as a prototype of a cognitive phenomenon ruled by a complex set of "rules" difficult to infer. Most of the work so far is based on the supervised inference of (subject-averaged) numerical ratings assigned to natural facial images. We present a novel approach based on the unsupervised (maximum entropy) learning of different subjects' preferences in the face-space. Interesting theoretical problems emerge within this approach, as the maximum entropy inference of data with a-priori correlations and constraints, or the Bayesian model selection between different maximum entropy models. We have applied these techniques to the analysis of an experiment in which several subjects are allowed to select their preferred variation of a reference face. The method unveils the essential subjectiveness and complexity of the criteria with which the facial images are selected, the crucial role played by the effective interaction between couples of facial coordinates, and their relative relevance in such a process.
Daniele Vilone
LABSS (Laboratory of Agent Based Social Simulation)
Institute of Cognitive Science and Technology,
National Research Council (CNR),
Via Palestro 32, 00185 Rome, Italy.
http://labss.istc.cnr.it/
it.linkedin.com/in/
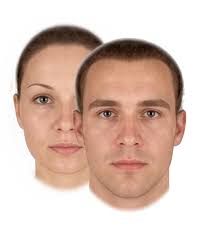